Sampling Time Series Data for Financial Insights
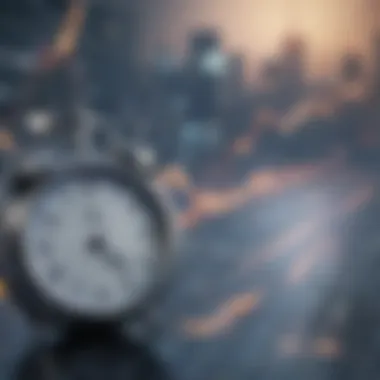
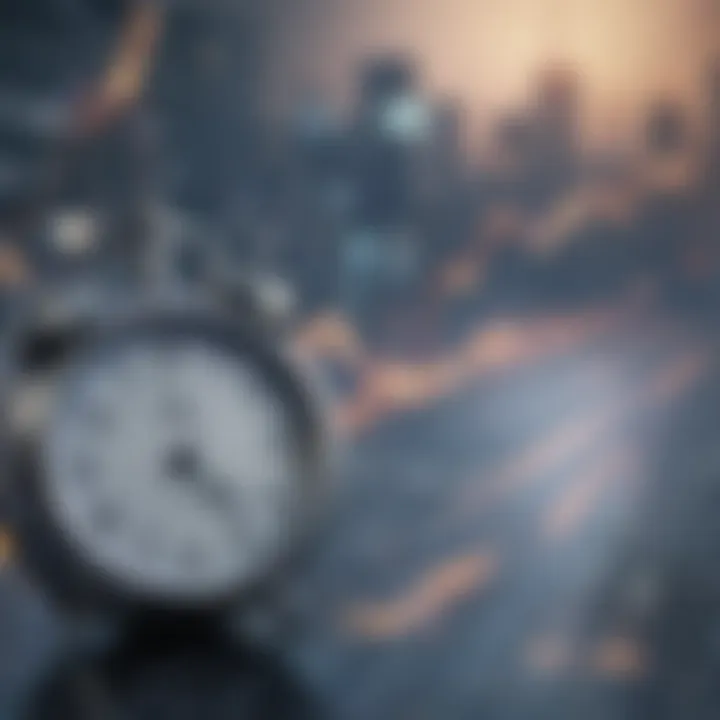
Intro
Understanding how to sample time series data is crucial, especially in finance, where informed decisions can lead to significant investments or losses. Timing is everything, and the accuracy of data collection plays a pivotal role in shaping the strategies that investors embark upon.
The approach to sampling time series data requires thoughtful consideration of various methodologies and their implications. This is not just about collecting data; it's about ensuring that the data accurately reflects the realities of the market over time.
Being able to navigate the subtleties will empower seasoned investors and newcomers alike to make well-informed choices. As we explore this intricate topic, we will demystify key concepts, dive into methodologies, and shed light on common pitfalls that accompany improper sampling.
Investment Definitions
To build a strong foundation, let's clarify some essential investment terms that underpin the realm of time series analysis.
Key Terms Explained
- Time Series: A sequence of data points recorded or measured at successive points in time, typically used to forecast future values based on historical trends.
- Sampling: The process of selecting representative data points from a larger dataset, crucial for conducting any significant analysis without getting lost in the noise of excessive data.
- Frequency: Refers to how often data points are collected over timeâdaily, weekly, monthly, or annually. The selection of frequency can deeply influence investment analysis.
- Bias: This originates from systematic errors in the sampling process. Recognizing various types of bias is vital to ensuring the integrity of analysis.
Common Investment Strategies
Investors apply several strategies that rely heavily on effective time series sampling, including:
- Technical Analysis: Investors often study historical price movements to identify patterns, using data from charts over time. Accurate sampling facilitates reliable trend identification.
- Statistical Arbitrage: This technique exploits pricing inefficiencies, heavily relying on sampled data to ascertain potential profit opportunities based on historical patterns.
- Value Investing: By analyzing historical performance and market indicators, investors seek undervalued assets, where a sound sampling approach can highlight opportunities others may miss.
Expert Advice
As we delve deeper into sampling methodologies, let's consider some strategies tailored for different types of investors.
Tips for Novice Investors
- Start small by focusing on a particular asset class. Keeping it limited helps ease the learning curve.
- Understand the significance of data frequency. Weekly data can provide different insights compared to daily data.
- Use visual aids like charts and graphs to help interpret your findings more readily.
Advanced Strategies for Seasoned Investors
- Experiment with non-linear sampling methods to tackle complex datasets that traditional sampling cannot handle effectively.
- Explore machine learning models for predictive analysis, leveraging diverse historical data inputs to increase forecasting accuracy.
- Regularly reassess your sampling methods to mitigate bias. Clarity in your initial data collection will save countless hours of reworking later.
Remember, the goal of good sampling is not merely to acquire more data, but to secure better insights that guide effective investment decisions.
Foreword to Time Series Data
Time series data represents a sequence of data points collected or recorded at specific time intervals. The very fabric of this field interweaves various aspects of analysis, especially within finance. Understanding time series data is not just an exercise in technicality; it's fundamental for anyone involved in financial marketsâfrom seasoned investors to aspiring analysts. The nature of this data allows one to track historical trends, identify cycle patterns, and predict future movements. It becomes abundantly clear that the significance of time-oriented data cannot be overstated.
There are several benefits that one can derive from a solid grasp of time series data:
- Trend Analysis: It permits a comprehensive view of how variables evolve over time. This is invaluable when assessing the performance of investments.
- Forecasting Capability: Time series aids in predicting future metrics based on historical data, making it a cornerstone for strategies in trading.
- Cyclical Insights: Seasonal patterns can be deciphered, facilitating informed decisions in timing market entries and exits.
In delving deeper into the nuances of time series data in financial analysis, one should remain cognizant that this approach also brings considerable challenges. The quality of data, the impact of outliers, and the possible occurrence of missing values are but a few hurdles present in this landscape. As such, mastering these intricacies requires a balanced mix of analytical skill and practical understanding.
Defining Time Series Data
At its core, time series data comprises data points indexed in time order. Unlike random data collection, time series data demands a structure, being collected at either consistent intervals or irregular frequencies. This data type is ubiquitous, found in everything from stock prices to economic indicators.
To clarify:
- Each data point in a time series conveys not just a figure but also a time stamp, allowing for observation and analysis of patterns across time.
- Given this arrangement, a researcher can consider how different variables interact over time, potentially uncovering invaluable insights for decision-making.
Significance of Time Series in Financial Analysis
When it comes to financial analysis, the utility of time series data is profound. Investors often rely on historical prices to inform their future choices. In an age where markets can swing wildly, understanding past behaviors can mean the difference between profit and loss.
Specifically, time series data can:
- Assist in Risk Assessment: By analyzing fluctuations in stock prices, analysts can gauge the volatility of an investment, understanding when it's prudent to pull back or make a move.
- Support Strategic Planning: Businesses utilize time series forecasts to manage inventories efficiently, ensuring that product supply aligns with demand cycles.
- Uncover Correlations: Observing time series data across various instruments can help articulate how different markets respond to macroeconomic shifts, providing strategic foresight.
"The past is a prologue to future gains; without understanding time series, investors walk a fine line between foresight and fortune loss."
In summation, embracing time series data is not only about analyzing numbers but also about appreciating their temporal contexts. As we press onward in this exploration, the intrinsic value of sampling in this realm will come to light, illustrating its powerful role in driving financial analysis to greater heights.
Foundations of Sampling
Sampling is a critical element in time series analytics, especially when it comes to making sound financial decisions. Understanding the foundations of sampling sets the stage for comprehending how we can extract meaningful insights from vast datasets. The importance of this topic lies in its ability to condense complex datasets into manageable insights, thus optimizing analysis while maintaining accuracy.
When we delve into the foundational concepts of sampling, we start to unrave how these techniques can lead to viable conclusions. For instance, by employing the right sampling methods, analysts can make informed predictions about market trends, gauge risks, and pinpoint opportunities. The effective utilization of sampling opens up a range of benefitsâit promotes efficiency, reduces cost, and enhances the potential for better decision-making.
Understanding Sampling Theory
Sampling theory encompasses the principles and strategies that guide us in selecting a subset of data from a larger population. At its essence, it addresses how and why we might choose to analyze a selected portion of data instead of the whole. The rationale behind sampling lies in practicality; gathering and examining every data point can be labor-intensive, time-consuming, and often unnecessary when patterns can be extrapolated from a representative subset.
Through sampling, we establish concepts like population parameters, sample statistics, and the relationship between them. Simply put, sampling allows us to make estimates about a broader group based on the analysis of a smaller, manageable one, thus making our predictions more efficient.
Types of Sampling Methods
Thereâs a variety of sampling methods, each suited for different scenarios, data characteristics, and analytical needs. Understanding these methods is essential for those serious about digging deep into time series analytics. Letâs break down some of the most prominent sampling methods:
Random Sampling
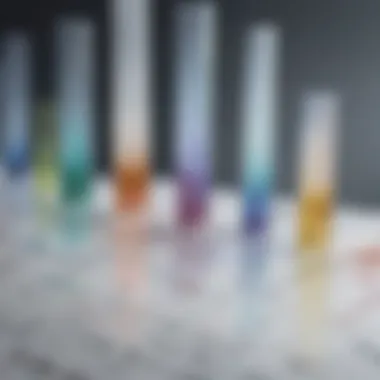
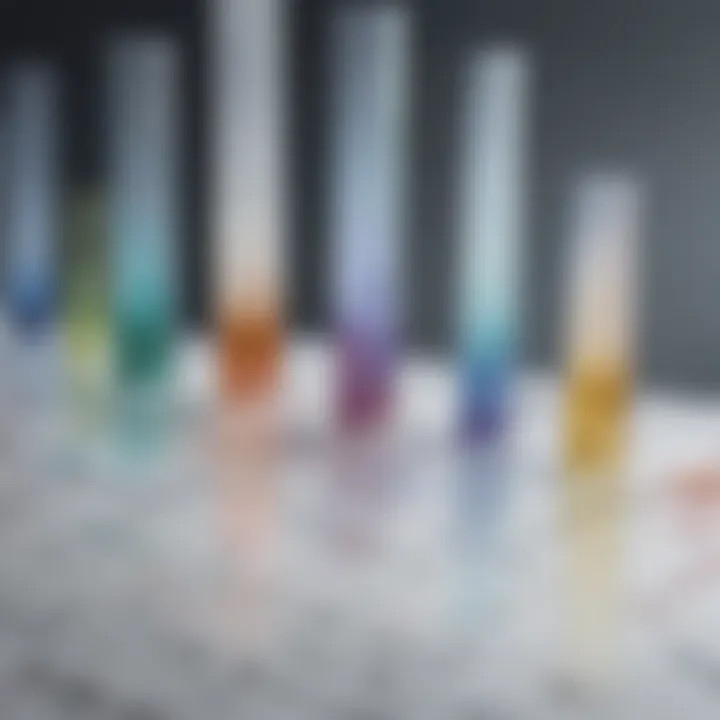
Random Sampling is like throwing darts at a board; every data point has an equal chance of being selected. The major strength of this approach is that it minimizes bias, allowing for a more authentic representation of the whole dataset. In the realm of financial analysis, random sampling is popular due to its simplicity and effectiveness. However, while itâs beneficial for gathering diverse data, if the sample size is not large enough or if certain critical segments are not included, it might lead to skewed insights.
Systematic Sampling
Systematic Sampling can be thought of as setting a rule; for example, you might decide to select every fifth data point from a sorted list. This method is beneficial because itâs straightforward to implement and often results in coverage of the population without the complicated nature of random selection. The unique feature here is its predictability. Yet, if thereâs an underlying pattern in the data that corresponds with the chosen interval, it could lead to misleading conclusions, so caution is critical.
Stratified Sampling
Stratified Sampling breaks down the population into smaller groups (or strata) that share similar characteristics. By choosing samples from each stratum, this method aims for precision. This technique is beneficial because it ensures representation across important subgroups, which is particularly essential in situations where certain segments hold weight in analysis, like different sectors within a financial landscape. However, the complexity of needing to define proper strata can sometimes be a handful.
Cluster Sampling
Cluster Sampling, unlike the previous methods, selects entire groups or clusters instead of individual points. It is particularly useful when dealing with geographically dispersed data. This technique is beneficial in cases where obtaining a complete dataset is challenging; by sampling clusters, analysts can gather insights without needing to reach out to every segment individually. A downside is that if the clusters are not homogeneous, the sample may not truly reflect the population.
Effective sampling can bridge the chasm between data abundance and insightful financial analysis.
In a nutshell, understanding these sampling methods is vital for navigating the complexities of time series data. Each method has its merits and potential pitfalls, making it crucial to choose the method best suited to the specific analytical context. Armed with these foundational concepts, financial analysts and decision-makers can embark on the journey of sampling with confidence.
Sampling Techniques for Time Series Data
Sampling techniques play a pivotal role in managing time series data, especially in financial domains where decisions hinge on accuracy and timeliness. The methods used to sample data not only affect the analysis outcomes but also influence the quality of forecasts and the reliability of those commanding investments. This section delves into various sampling techniques crucial for effective financial analysis, highlighting their unique benefits and considerations.
Choosing Appropriate Sampling Techniques
When it comes to selecting the right sampling method for time series data, one must consider the nature of the data as well as the specific objectives of the analysis. Several factors come into play:
- Nature of Data: Is the data continuous, discrete, or irregular? Understanding the attributes of the dataset is essential.
- Objectives: What are the analysis goals? Are you forecasting future values, detecting anomalies, or assessing seasonal patterns?
- Resource Constraints: Time and computational resources can also limit which methods are viable.
- Data Integrity: Ensuring the sampled data is representative of the whole data set is key in avoiding biases.
Each choice has its pros and cons, and what works for one type of analysis in finance might not suit another. Thoroughly assessing these elements will aid in landing on the most appropriate technique.
Fixed Frequency Sampling
Fixed frequency sampling is a method where data is collected at regular intervals. This technique is particularly beneficial in financial analysis due to its straightforward implementation and consistency. Imagine taking stock prices daily at closing time; this method allows for the evaluation of trends over time without the chaos of random sampling interruptions.
Some significant points to keep in mind include:
- Time Series Stability: Regular intervals aid in maintaining consistency which is crucial in evaluating historical trends.
- Seasonal Effects: This technique helps in spotting seasonal variations since data collected produces a clear picture over defined periods, improving the chances of accurate seasonality detection.
- Simplicity: The ease of implementation makes fixed frequency sampling a go-to for many analysts, particularly when initial resources are limited.
However, one must be cognizant of potential pitfalls, such as overlooking abrupt changes in trends outside the regular collection times, which could misrepresent the overall financial landscape.
Irregular Sampling and Its Implications
Unlike its fixed counterpart, irregular sampling does not adhere to a set schedule for data collection. This method might seem messy, but itâs often reflective of real-world scenarios. For instance, trading volume spikes during certain events, leading to data points that are not uniformly distributed across time.
Here are some considerations regarding irregular sampling:
- Realism: It mirrors the unpredictable nature of financial markets, allowing for a more authentic representation of the data.
- Data Gaps: However, irregularities can lead to missing insights and patterns unless handled adeptly. Analysts must be prepared to fill these gaps using imputation techniques or re-weighting methods.
- Complexity in Analysis: Dealing with irregular data requires sophisticated models. It can lead to increased computation time and demands for robust statistical approaches.
Overall, while irregular sampling may introduce challenges, it can also enrich analyses by encapsulating the dynamics of market changes that fixed sampling would gloss over.
Success in the realm of financial analysis hinges significantly on understanding and applying the right sampling techniques for time series data, as each choice carries inherent implications for the outcomes.
Challenges in Sampling Time Series Data
Sampling time series data is often not a walk in the park. It comes with its own set of hurdles that can significantly impact analysis and decision-making. Understanding these challenges is crucial for anyone looking to delve into financial data or make investment choices. Grasping the nuances behind these issues equips one to navigate the complex maze of time series sampling with greater confidence and precision.
Data Quality Issues
When diving into time series data, the phrase "garbage in, garbage out" rings especially true. The quality of the data being sampled is fundamental. If the original data is riddled with inaccuracies, the resultant analytics will likely leave much to be desired. Here are some common data quality issues:
- Noise in Data: Time series data often contains random variations or anomalies that do not represent the underlying trend. This noise can obscure meaningful insights.
- Outliers: Unexpected data points that deviate significantly from the rest can skew analysis, leading to faulty conclusions. Identifying and handling these outliers is critical.
- Data Consistency: For time series to be effective, the data must be consistent over time. Inconsistencies can arise from varying data collection methods or changes in the data source.
Ensuring data quality demands rigorous validation processes and a keen eye for detail. Think of it as building a house on a shaky foundation; any issue at this stage could collapse the whole structure of your analysis.
Handling Missing Data
Missing data is the thorn in the side of time series sampling. Whether itâs caused by data entry errors, system failures, or other unexpected mishaps, the absence of data can introduce bias and distort the results. Here are some strategies to handle missing data effectively:
- Data Imputation: This involves filling in missing values based on available data. Techniques like mean imputation, median imputation, or more advanced methods, such as predictive modeling, can be effective depending on the context.
- Deletion Methods: Sometimes, it may be best to simply exclude missing data points â but this can lead to loss of information and reduced sample size. Care must be taken to ensure that this does not introduce bias into the quality of the analysis.
- Model-Based Approach: Incorporate models that can handle missing data naturally, like certain time series forecasting methods that do not require a complete dataset.
Thereâs no one-size-fits-all solution when dealing with missing data. It's about strategically weighing which option aligns best with the investment analysis at hand.
Sampling Bias: Understanding the Risks
Sampling bias arises when certain elements of the population are systematically favored over others during the sampling process. For time series data, this can lead to skewed interpretations that misrepresent the actual situation. Consider these points regarding sampling bias:
- Over-representation of Certain Periods: If sampling favors certain time frames (like economic booms vs. downturns), it may paint an unrealistic picture of performance or risk.
- Selection Bias in Data Sources: Choosing data from only specific platforms or channels can exclude essential segments of the market. This can lead to incomplete analyses and decisions based on anecdotal evidence.
- Temporal Bias: Time-related biases arise when samples are collected over different time periods which may not be comparable. For example, analyzing behavior during extreme market conditions may not reflect typical patterns.
It's essential to be alert and mindful of these biases, ensuring that the sampling method aligns with the true characteristics of the entire dataset. Effective time series analysis hinges on recognizing these biases and mitigating their influence on overall insights.
"Recognizing the limitations and challenges inherent in sampling time series data is the first step toward effective analysis."
Understanding these challenges is not just helpful; it's vital. Each obstacle offers an opportunity for better methodologies, ultimately leading to sharper, more informed financial insights.
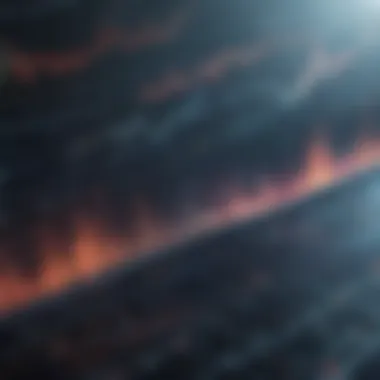
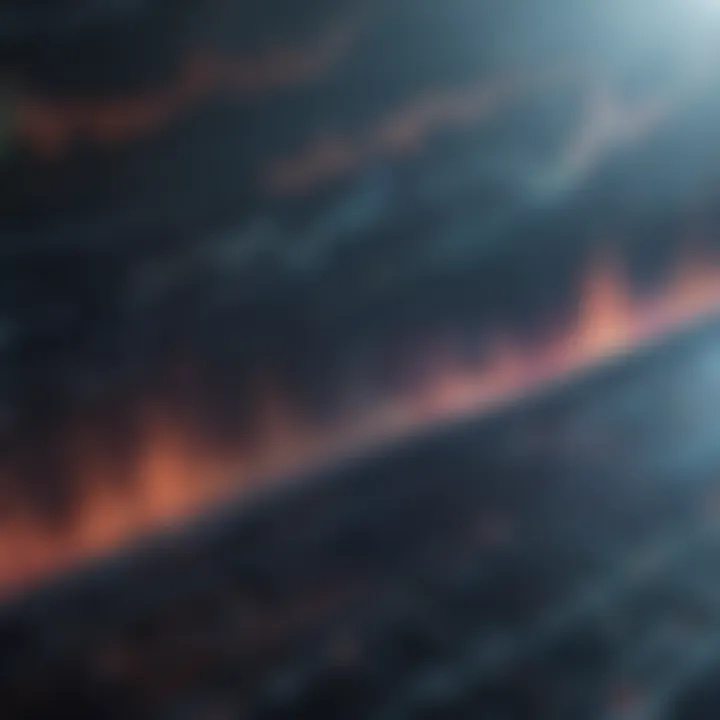
Statistical Methods in Time Series Sampling
Statistical methods are the backbone of sampling in time series data. They provide the tools and frameworks necessary for making sense of complex and often voluminous data. In financial analysis, where every decision can result in significant gains or losses, having a robust set of statistical methods available is paramount. These methods not only help in structuring the data effectively but also guide analysts in making informed predictions about future trends based on sampled observations.
A key benefit of employing statistical methods in time series sampling is that they enable analysts to extract meaningful insights from large datasets. By understanding patterns such as seasonality and volatility, financial professionals can better anticipate market movements. Additionally, these methods allow for the quantification of uncertainty, making it possible to gauge the reliability of forecasts.
Considerations surrounding statistical methods also hinge on accurately recognizing the nature of the dataâwhether it's stationary or non-stationary, for instance. This understanding is crucial because it determines which methods will yield valid results. Therefore, a solid grasp of the underlying characteristics of time series data cannot be overstated.
Estimation Techniques
Estimation techniques in time series sampling involve statistical approaches that help in approximating unknown parameters within a dataset. These techniques can significantly influence the outcome of any analysis. Ordinary least squares, for example, offers a simple yet powerful method for estimating relationships within data. However, in time series contexts, more tailored methods, such as Maximum Likelihood Estimation (MLE), are often preferred. This is largely due to their capability to handle the unique challenges presented by time-dependent data.
Additionally, estimators can also be categorized based on their bias and variance properties. Biased estimators may yield results that are systematically off from the true parameter, while unbiased estimators strive for accuracy. Analysts often find themselves balancing these aspects when deciding on which estimation method best fits their needs.
Forecasting Methods for Sampled Data
Forecasting methods translate historical time series data into future predictions. These techniques are invaluable for investors and financial advisors as they lay the groundwork for strategy development.
ARIMA Models
Autoregressive Integrated Moving Average (ARIMA) models excel in capturing different components of a time series datasetânamely, the autoregressive part, moving average part, and the integrated part. The contribution of ARIMA models to financial analysis cannot be understated; they are particularly adept at handling univariate time series forecasting problems. A notable characteristic of ARIMA is its flexibilityâanalysts can modify it to account for trends and seasonality, thereby tailoring the model to fit a wide variety of datasets.
The unique feature that sets ARIMA apart is its incorporation of differencing. This method is employed to achieve stationarity in the data. While the model can be particularly powerful, it does come with some limitations, such as requiring a relatively large dataset for accurate estimations and the need for the analyst to identify appropriate parameters, which can at times be complicated.
Exponential Smoothing
Exponential smoothing is another forecasting method that has gained popularity, especially because of its simplicity and effectiveness. One of the key characteristics of exponential smoothing is its ability to weigh recent observations more heavily than older ones. This feature is beneficial in fast-paced financial environments where recent trends are often more indicative of future movements.
Moreover, the method can be adjusted through its various formsâsimple, double, or triple exponential smoothing. Each form caters to different data characteristics. However, the downside of this approach lies in its assumption that past data will continue to behave in a similar manner moving forward. This might not hold true in volatile markets, leading to potentially skewed forecasts.
Practical Applications of Sampling in Finance
Sampling time series data stands as a cornerstone in financial analysis, enabling investors to distill vast datasets into manageable insights. It's not just about collecting numbers; itâs about pulling back the curtain to understand underlying trends, behaviors, and risks. As such, practical applications of sampling in finance encompass a wide array of activities, from risk management to performance evaluation, all crucial for informed financial decision-making.
Risk Management and Sampling
Risk management in finance deals with identifying, assessing, and mitigating potential losses arising from a variety of sources. Sampling plays a pivotal role in this realm. By using targeted samples from time series data, financial analysts can forecast risk more accurately. For instance, consider a financial institution that needs to assess the risk of loan defaults. Instead of examining the entire database, which could be overwhelming, a well-structured sample might reveal patterns of default that are not immediately visible in the larger dataset.
Furthermore, sampling helps in stress testing â an essential process for evaluating how a portfolio could withstand adverse market conditions.
- Data-driven insights: A smaller, well-chosen sample can yield insights that are not apparent from random selections.
- Efficiency: Sampling reduces the time it takes to compute risk metrics, thus allowing quicker responses to changing market conditions.
Investment Performance Analysis
When it comes to evaluating investment performance, sampling provides a focus on significant datasets that could skew results if holistic analysis was applied. Financial analysts can look at representative samples over various periods, allowing them to evaluate how an investment has performed under different market conditions.
Investors might use samples to calculate metrics such as the Sharpe ratio, which assesses risk-adjusted returns. This helps in comparing various investments on an equivalent footing. In this context, historical time series data sampled effectively can shine a light on:
- Temporal performance trends: It reveals how investment performance has changed over time, considering economic cycles.
- Contextual performance analysis: Analysts can position returns in relation to the greater market movement, providing a baseline for assessing future performance.
Accurate sampling leads to richer insights, ultimately guiding investment decisions that can help maximize returns while minimizing risk.
Portfolio Diversification Strategies
Last but not least, portfolio diversification stands as a fundamental principle in investment strategies â spreading risk across various financial instruments to reduce the impact of any single asset's downturn. Sampling plays a crucial role in the optimization of diversification strategies. Analysts can draw samples from diverse asset classes, measuring their historical correlations and volatilities.
Through exploratory sampling, an investor can identify the right mix of assets, balancing potential rewards against risks effectively.
Points to consider include:
- Optimization of asset allocation: Sampling practices can help determine which assets work well together, reducing exposure to risks that impact similar investments simultaneously.
- Performance against benchmarks: With sampled data, portfolio managers can adjust holdings if a particular strategy isnât achieving desired outcomes compared to relevant benchmarks.
Investing isnât just about buying and holding; itâs about balancing, adjusting, and continuously refining after evaluating sampled data.
"Sampling time series data is akin to possessing a magnifying glass; it allows investors not only to notice the obvious elements but also to spot the nuances that may escape even the most experienced eyes."
In summary, the practical applications of sampling in finance are both numerous and significant. They offer an efficient pathway for insights across risk management, performance analysis, and diversification strategies, essential for anyone seeking to navigate the complexities of financial markets.
Tools and Software for Sampling Time Series Data
In the realm of sampling time series data, itâs crucial to have the right tools and software in your toolkit. With financial analysis becoming ever more complex, the variety of sampling methods needs to be managed and executed with precision. This section explores various software options available and how they streamline the sampling process, paving the way for more informed investment decisions.
Statistical Software Overview
R and Python for Time Series Analysis
R and Python are two heavyweight contenders in the arena of time series analysis. They shine in their capacity to handle large datasets, make intricate calculations, and provide visualizations that simplify understanding complex data.
In particular, R has a rich ecosystem of packages specifically designed for statistical modeling and time series analysis, such as , , and . The main strong point of R lies in its statistical prowess, enabling users to carry out sophisticated analyses almost effortlessly.
Python, on the other hand, has surged in popularity due to its versatility and ease of use, especially with libraries like , , and . Its community is vibrant, offering abundant resources and support, making it a solid choice for beginners and seasoned analysts alike. However, one unique feature of Python is its ability to integrate with other platforms easily, enhancing its functionality for web scraping and data manipulation.
While R is often favored for deep statistical tasks, Python steals the show when it comes to broader data processing needs. Thus, choosing between R and Python largely hinges on the specific requirements of your analysis and your personal comfort level with each programming language.
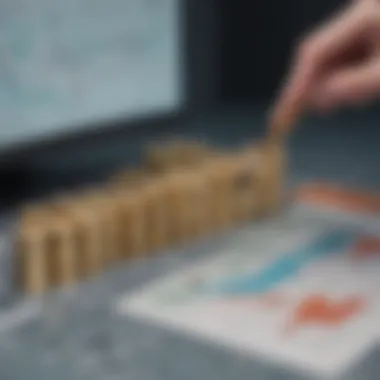
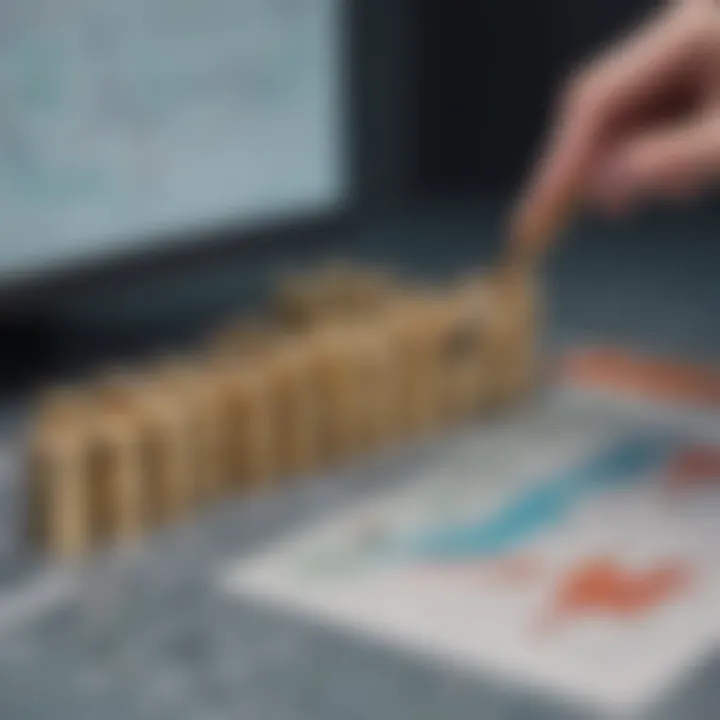
Excel for Financial Data Sampling
Excel remains a titan in the business and finance worlds, often regarded as a go-to tool for sampling financial data. Its simplicity and ease of use make it a widely adopted choice. Many analysts leverage Excel for quick data manipulation, creating pivot tables, and conducting basic statistical analyses.
One of Excel's key characteristics is its accessibility; most users are somewhat familiar with its features, making it easy to hit the ground running. Specifically, tools like data visualization charts, and built-in formulas can help users quickly draw insights from their data without delving into coding.
That said, while Excel is incredibly useful for simple tasks, it does have limitations. Handling very large datasets can lead to performance issues, and its statistical capabilities are not as robust as those found in dedicated statistical software like R and Python. In sum, Excel is a great entry point but may not suffice for more complex financial analyses.
Choosing the Right Software for Your Needs
Selecting the appropriate software for sampling time series data involves understanding the specific requirements of your analysis. Here are some considerations that may influence your decision:
- Complexity of Analysis: If the analysis demands intricate statistical methods, R might be more suitable.
- Data Volume: For large datasets, consider Python's capabilities and efficient handling of big data.
- Learning Curve: If you're new to data science, Excel could serve as a sufficient starting point, while R and Python may have steeper learning curves.
- Long-term Usage: Think about whether you're looking for a one-off analysis or a software solution for ongoing projects; this could sway you towards more robust platforms.
Ultimately, the right software should align with your analytical goals, skillset, and the specific challenges you're facing in time series data sampling.
Case Studies on Time Series Data Sampling
Understanding the practical implications of sampling time series data is crucial in a fast-paced financial environment. Through case studies, we can illuminate the real-world applications of theory and methodology, offering tangible illustrations that heighten comprehension. These examples serve not just to highlight successful strategies ; they also identify pitfalls and areas for improvement. This nuanced perspective ensures that both investors and analysts appreciate the intricacies involved in their decision-making processes.
Successful Applications in Investment Analysis
Investment analysis demands precision and insight. Case studies exhibit how methodical sampling can impact investment returns and risk management. For instance, the infamous example of the Dot-Com Bubble illustrates how sampling helped analysts identify patterns in stock prices across tech companies.
By narrowing down their focus to a representative subset of firms, analysts could assess growth potential without being misled by anomalies caused by outliers or market euphoria. The selected samples, based on criteria such as market capitalization and earnings growth, allowed for a more informed investment strategy.
Another compelling case is seen with the housing market analysis prior to the financial crisis of 2008. Researchers employed systematic sampling techniques to gauge trends in housing prices across various segments. By identifying neighborhood-level data, they could forecast potential declines more accurately than relying on broader market indices. Such targeted analysis enabled investors to adjust their portfolios ahead of tumbling prices, showcasing the value of adequate sampling.
âDiving into case studies not only clarifies the benefits of sampling but also emphasizes awareness of the diverse sampling methods in play.â
Lessons from Failed Sampling Approaches
Studying failures is equally critical. The energy crisis of the 1970s provides a vivid example where poor sampling led to flawed predictions. Analysts relied on limited monthly data from a handful of oil companies, which led to a misunderstanding of demand and supply dynamics. The narrow focus failed to account for global market shifts, resulting in miscalculations that severely affected investment portfolios.
Moreover, the 2000 presidential election, which was marred by sampling errors, highlights the dangers inherent in poorly executed data collection. Pollsters missed the mark when they under-sampled certain demographics, leading to an expectation that did not match the actual voting results. Investors, who often rely on polls for market sentiment, can learn from these misstepsâacknowledging the critical nature of a representative sample.
Epilogue of Case Studies
Through these case studies, it becomes evident that understanding and applying sampling techniques are pivotal for making sound investment decisions. Successful applications reveal the benefits of rigorously tested methodologies, while failures underscore the importance of comprehensive data collection. As investors and financial advisors navigate the complexities of time series data, these real-world instances can act as guiding lights, illuminating pathways to informed strategies and reinforcing the necessity for precision in sampling.
Emerging Trends in Time Series Sampling
In the realm of financial analysis, staying ahead of the curve is crucial, especially when it comes to sampling time series data. As markets evolve and the volume of available data increases dramatically, emerging trends play an essential role in shaping methodologies and strategies for effective analysis. Understanding these trends can significantly influence investment decisions, risk assessment, and overall financial performance. This section focuses on two primary elements: the role of big data and the integration of machine learning into time series analysis.
The Role of Big Data in Sampling
Big data has changed the game for financial analysts and investors alike. The sheer volume of data now available offers an unprecedented opportunity to sample a wider range of time series, thereby providing richer insights. However, with this opportunity comes a slew of challenges that practitioners must navigate.
- Data Variety: Unlike traditional data, big data encompasses diverse formats from various sources, including social media, transaction records, and market indicators. This multiplicity requires adaptive sampling methods that can accommodate different data types.
- Data Velocity: The speed at which data flows in from multiple channels is staggering. Timeliness is key in financial markets, necessitating real-time sampling techniques to keep pace. This influences the way analysts approach data collection and preprocessing.
- Data Veracity: With the increase in data volume, ensuring its accuracy and relevance becomes paramount. Sampling strategies must prioritize data quality to avoid biases that could skew results and lead to faulty conclusions.
Embracing big data is not merely about collecting vast quantities of information; it involves refining methods to analyze and sample effectively. For instance, techniques such as parallel processing and cloud computing can enhance data handling capabilities, allowing for more intricate and timely analyses.
"In the world of finance, the ability to leverage big data for strategic sampling may well determine the winners and losers."
Machine Learning and Time Series Analysis
The integration of machine learning into time series analysis is another noteworthy trend that stands to revolutionize the sampling landscape. Machine learning algorithms help automate and optimize the sampling process, providing a level of analysis that traditional methods may fail to deliver.
- Predictive Modeling: Machine learning can facilitate the creation of predictive models, identifying patterns within sampled data that may not be immediately apparent. For instance, algorithms like ARIMA or LSTM can predict future price movements based on historical data, aiding investment strategies.
- Adaptive Sampling: One of the crucial advantages of machine learning lies in its ability to adapt sampling techniques based on emerging data trends. Rather than relying on static sampling methods, practitioners can employ algorithms that adjust dynamically, taking advantage of real-time insights.
- Anomaly Detection: With the ability to process large datasets quickly, machine learning can identify outliers or anomalies in time series data that require further investigation. Recognizing these irregularities promptly aids in risk management and decision-making.
As these trends continue to develop, they underscore the necessity for investors and financial analysts to keep pace with technological advancements. The synergy of big data and machine learning offers powerful tools to refine the sampling of time series data, ultimately leading to better-informed financial decisions.
End
The conclusion serves as a pivotal point in this article, encapsulating the myriad themes that have traversed the landscape of sampling time series data. This section synthesizes crucial insights, reinforcing the essential role that sampling plays not only in financial analysis but in broader contexts. By dissecting the complex methodologies and challenges tied to sampling practices, we highlight how a well-structured approach can influence investment decisions and strategies endorsed by financial advisors.
A primary benefit derived from the culmination of this exploration is the enhanced understanding of how various sampling techniques can serve different analytical goals. For example, the distinction between fixed frequency sampling and irregular sampling reveals how the choice of method can impact the accuracy and reliability of financial forecasts. Furthermore, acknowledging potential biases enhances the astuteness of investors and analysts alike, facilitating more informed, data-driven decisions.
What stands out is the intrinsic link between data sampling and the quality of insights garnered from time series analysis. As financial environments grow increasingly complex, the importance of precise and rigorous sampling protocols cannot be overstated. This conclusion stresses that dismissing fundamentals or oversights in sampling may lead to misguided financial strategies, underlining the necessity for vigilance and critical analysis in every sampling endeavor.
"In the world of finance, data is not just numbers; it's the pulse of market sentiment, and sampling correctly is how we hear that pulse clearly."
It is through this lens of understanding that the article catalyzes further inquiry and application, urging readers to employ these insights adeptly in their respective fields.
Summarizing Key Insights
Summarizing the key insights from the exploration, we can delineate some critical aspects that directly contribute to the mastery of sampling time series data:
- Importance of Sampling Techniques: Different methods like random, systematic, and stratified sampling each have unique advantages that align with specific objectives in financial analysis.
- Navigating Data Frequency: The frequency at which data is sampled fundamentally alters the outcomes and reliability of analyses, requiring keen attention to temporal variables.
- Awareness of Biases: Familiarity with sampling biasesâwhether they stem from wrong assumptions about data distribution or the choice of sampling methodsâcan substantially mitigate risks.
- Application of Statistical Tools: Utilizing statistical software such as R and Python alongside Excel can enhance data manipulation and facilitate more robust analysis across varied contexts.
These insights reinforce the necessity of informed decision-making processes when engaging with financial data and underline the impact of effective sampling in shaping investment practices.
Future Directions for Research
Looking ahead, several avenues for future research emerge from this comprehensive overview of sampling time series data:
- Integration of Big Data: As the financial landscape continually evolves, integrating big data methodologies alongside traditional sampling perspectives promises enhanced predictive capabilities and investment strategy formulation.
- Advancements in Machine Learning: Exploring machine learning techniques to refine sampling methods presents an exciting frontierâleveraging algorithms may uncover patterns not easily discernible through conventional analyses.
- Interdisciplinary Approaches: Enriching time series analysis through interdisciplinary studies could yield innovative frameworks. For instance, insights from behavioral finance may inform sampling strategies that account for human elements influencing market movements.
- Experimental Designs in Sampling: Future exploration might include experimental designs focusing on how various sampling methods can be tested in real market scenarios, fostering a deeper understanding of their practical outcomes.
These research directions aim not only to contribute to theoretical knowledge but also to empower practitioners with actionable strategies in the frequently changing dynamics of financial markets.