Mastering Time Series Graphs for Financial Analysis
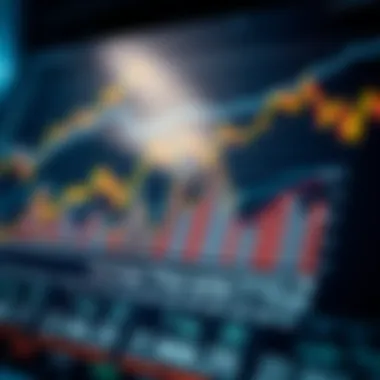
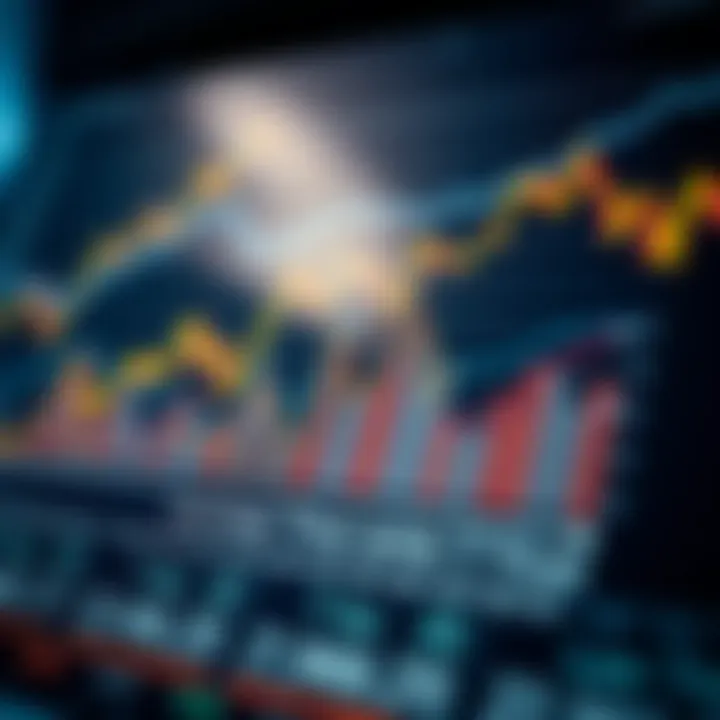
Intro
In financial circles, the ability to interpret data through time series graphs has become increasingly vital. These graphs represent data points sequenced over time, enabling investors to pinpoint trends, catches anomalies, and make educated decisions. Whether you are an investor just starting out or a seasoned financial analyst, a thorough comprehension of these visual tools enhances your financial acuity.
In this guide, we will illuminate the sheer potential of time series graphs in financial analysis. From foundational definitions and terminology to sophisticated strategies for implementation, this article aims to broaden your understanding and enhance your decision-making prowess in the financial realm.
Let’s delve into the core concepts to set the stage for our analysis.
Understanding Time Series Graphs
Understanding time series graphs is crucial for anyone involved in financial analysis. These visuals capture data across time intervals, allowing investors and analysts to gain insight into trends, patterns, and anomalies. By effectively using time series graphs, one can enhance their financial decision-making capabilities.
The beauty of time series graphs lies in their ability to present vast amounts of information in an accessible format. When professionals examine stock prices or interest rates, they are not just looking at numbers; they are decoding a story that those numbers tell. One can spot upward trending movements that indicate a healthy market, or perhaps more unsettling, shifts that hint at instability. Thus, interpreting these graphs is not merely a technical endeavor; it’s an art of understanding the implications behind the data.
Definition and Basics
A time series graph is a simple yet powerful tool that showcases data points plotted chronologically. In essence, it paints a picture of how a specific variable changes over time. This format allows for the comparison of various time frames, whether analyzing daily stock prices or yearly GDP growth. The primary axes of these graphs usually consist of:
- X-axis: Represents the time intervals, which could be days, months, or years.
- Y-axis: Displays the value of the variable being measured (such as stock prices or economic indicators).
A basic understanding of the concepts behind time series graphs can serve investors well as they try to make sense of market behaviors. Whether discussing a bullish phase or a bearish downturn, these visualizations help contextualize financial realities.
Historical Context
The concept of time series analysis can be traced back several centuries, with roots in early statistics and econometrics. However, it wasn’t until the 20th century that time series methodologies began to gain significant traction in economic studies.
Historically, individuals like George E.P. Box and Gwilym M. Jenkins laid the groundwork for many models still in use today, namely the ARIMA (AutoRegressive Integrated Moving Average) models. Their publication, Time Series Analysis: Forecasting and Control, brought a systematic approach to analyzing temporal data.
In practice, time series analysis has undergone a renaissance thanks to advancements in computing and data visualization technologies. Today, financial analysts harness these tools to predict future market behaviors and refine strategies. As technology evolves, the relevance and application of time series graphs continue to flourish, shaping financial decision-making worldwide.
"The future cannot be predicted, but futures can be invented." – Dennis Gabor
Understanding these graphs and their historical evolution can lay a foundation for appreciating their current utility in financial contexts. Investors, analysts, and financial advisors must recognize the significance of looking back at trends to forecast the future.
Types of Time Series Data
Time series data, in the realm of financial analysis, is a critical tool that allows analysts to identify opportunities and risks. Understanding the types of time series data is paramount, as it directly influences the decision-making process in financial contexts. Not all time series are created equal; recognizing the distinctions among different types can lead to more informed strategies and outcomes.
Continuous vs. Discrete Data
When discussing time series data, one must first grapple with the basic classifications: continuous and discrete data. Continuous data can take any value within a range, making it incredibly versatile. For example, stock prices can fluctuate continuously, reflecting live market conditions. This flexibility allows for detailed analysis, driving home the importance of minute-to-minute changes — which is crucial in high-stakes trading.
On the other hand, discrete data presents distinct values, often representing a countable number of observations over set intervals. Think of the number of trades executed in a day or monthly interest payments. While this type of data may not be as fluid as its continuous counterpart, it still holds valuable insights, particularly for trend analysis over defined periods.
Overall, recognizing the nuanced differences between these two types of data is essential for financial analysts. They must choose the right type based on what they aim to achieve.
Financial Time Series Data
Diving deeper, let’s explore financial time series data specifically. This category usually includes critical data types such as stock prices, interest rates, and exchange rates. Each of these data points carries its unique story, illuminating aspects of market behavior and economic trends.
Stock Prices
Stock prices are the lifeblood of equity markets, serving as barometers for company health and investor sentiment. Each price reflects myriad factors, from earning reports to market speculation, making them a favorite for analysts. The key characteristic of stock prices is volatility; they can shift dramatically within seconds based on news or events, which provides both a risk and an opportunity for savvy investors.
A unique feature of stock prices is their tendency to display trends over time. Analysts often utilize moving averages to smooth out daily fluctuations, enabling clearer insights into longer-term price movements. The advantage of closely monitoring stock prices is the ability to make quick trades, leveraging small price changes. However, the downside lies in their unpredictability, which can lead to significant losses if not managed properly.
Interest Rates
Interest rates play a pivotal role in economic health and affect virtually all aspects of finance. They dictate the cost of borrowing and the return on savings, influencing consumer behavior and investment decisions. What defines interest rates is their responsiveness to economic indicators; they often adjust based on inflation and monetary policy.
Interest rates are particularly beneficial as they can help project future financial landscapes through economic forecasts. Unique here is their dual nature; while low rates can stimulate borrowing and spending, high rates may suppress these activities. Thus, analysts must navigate the fine line between using interest rates as leverage and being cautious of rate hikes that could stifle growth.
Exchange Rates
Exchange rates are another key player in the world of financial time series data. These rates indicate how much one currency is worth in relation to another and are crucial for international trade and investment. The key characteristic of exchange rates is their liquidity; they are traded continuously in the foreign exchange market, subject to real-time fluctuations based on geopolitical events, economic indicators, and market speculation.
One unique aspect of exchange rates is their impact on multinational corporations. A favorable exchange rate can enhance profitability for companies operating abroad, while adverse shifts can lead to significant financial risks. The primary advantage of tracking exchange rates is the ability to hedge against currency fluctuations, but it can also be a double-edged sword when unexpected economic changes occur.
Understanding these types of financial time series data — stock prices, interest rates, and exchange rates — enriches the overall analysis. By grappling with their unique attributes, analysts can better navigate the complexities of financial markets, making more sound investment decisions and strategic moves.
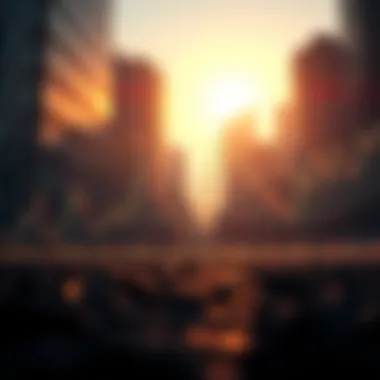
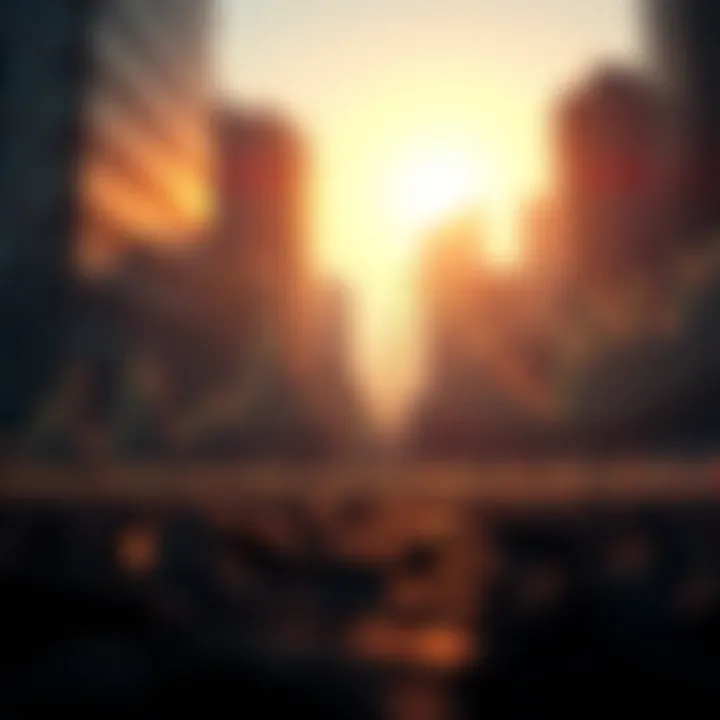
Components of Time Series Analysis
Understanding the components of time series analysis is pivotal for any financial analyst or investor aiming to make sense of data over time. The multifaceted nature of time series data encompasses not only the surface trends but also underlying forces that influence data behavior. This exploration reveals how awareness of trends, seasonality, cyclic patterns, and irregular variations can empower analysts to draw insightful conclusions, ultimately leading to better financial decisions.
Trend
The trend in a time series is akin to a broad current that defines the direction in which the data is headed over an extended period. Recognizing whether the trend is upward, downward, or horizontal forms the backbone of predictive analytics. Investors can discern whether a stock is gaining momentum, stabilizing, or diminishing over time. For instance, if a company's stock price consistently rises over several quarters, it may justify investing for the long haul.
But it's not just about identifying the trend—it's critical to understand its persistence and strength. A slight upward movement might not signal a strong bullish market if influenced by temporary factors. A robust trend reveals itself through consistent data points bolstered by substantial trading volume or favorable market conditions.
Seasonality
When discussing seasonality, think of it as the rhythm of the market. Certain financial datasets exhibit predictable fluctuations based on recurring events—like holiday shopping sprees impacting retail sales or quarterly earnings that sway stock prices. For instance, an airline might see a spike in revenue during summer travel periods.
In financial analysis, recognizing seasonal patterns is essential for accurate forecasting. Failure to consider seasonality could lead to inflated expectations or misguided strategies. Analysts often employ different techniques like Seasonal Decomposition of Time Series to separate these regular patterns from the overall trend. The key to making savvy financial decisions often lies in these seasonal fluctuations, acting as a compass for strategizing in investment.
Cyclic Patterns
Cyclic patterns, in contrast to seasonality, represent longer-term fluctuations that do not adhere to a fixed time frame. These cycles can resonate like the waves of an ocean, influenced by various economic indicators such as booms and recessions. For example, an economic cycle might span several years, where an industry experiences growth followed by a downturn due to shifts in consumer demand, regulatory changes, or technological advancements.
Understanding cyclic patterns allows analysts to anticipate extensive market movements and adapt strategies accordingly. Recognizing when an industry is entering a bull or bear market can profoundly affect investment choices, balancing expectations against potential market shocks.
Irregular Variations
Irregular variations, sometimes called noise, can be the wild card of time series analysis. These unpredictable fluctuations may arise from unforeseeable events—like natural disasters, political upheaval, or other sudden shifts in market dynamics. For example, a pandemic can dramatically alter consumer behavior and disrupt supply chains, leading to a sharp decline in business performance.
It’s crucial to factor in these irregularities, as they can skew the interpretation of data trends and patterns. While they may not fit neatly into the categories of trend, seasonality, or cyclic behavior, analysts should remain aware of their potential impact on the data, including how they might mask genuine signals or create illusions of stability.
Recognizing irregular variations is essential, as they remind us that not all market movements are predictable or explained by historical data.
In summary, the components of time series analysis—trend, seasonality, cyclic patterns, and irregular variations—are integral to making informed financial decisions. By dissecting these elements, analysts can find clarity amid the complexities of financial data, leading to a more nuanced understanding of market behavior.
Constructing a Time Series Graph
Creating a time series graph requires a balanced mix of technical skill and an understanding of the financial data at hand. It’s not just about plotting points on a chart; it’s about crafting a visual narrative that tells the story of those data points over time. An effective graph can highlight important trends and patterns that might not be evident just by looking at raw numbers.
One crucial element of constructing these graphs involves data collection techniques. The integrity of your results starts here. Good data leads to insightful analyses. Consideration must be given to how the data is collected, cleaned, and prepared for analysis. Additionally, the tools used for graphing must be relevant and efficient, directly influencing the clarity and visual quality of the graph. The following sections delve into the various tools that can be leveraged for graphing, such as Excel, Python libraries, and specialized statistical software.
Moreover, adhering to graph design principles is vital. A well-designed graph not only conveys information but does so clearly and attractively. Elements like color, scale, and labeling all play significant roles in how the viewer interprets the data. By mastering graph construction, analysts can transform complex financial data into intuitive graphical representations that drive better decision-making in financial analysis.
Data Collection Techniques
When it comes to financial time series data, collection is half the battle. Techniques may vary, but a few key methodologies stand out. First off, there’s surveys; gathering data directly from stakeholders can be insightful. For example, investor sentiment can be gauged through targeted questionnaires, providing qualitative time series data. Another popular method is to utilize financial databases, like Bloomberg or Yahoo Finance. These platforms gather massive amounts of data automatically using APIs, saving analysts significant time.
It’s also essential to ensure that the data is both reliable and relevant. Failing to prep these details often means grappling with inaccurate conclusions down the line. When you’re working with stock prices, ensuring that the data reflects the correct trading hours and includes key events like stock splits or dividends will enhance the temporal accuracy of your time series.
Tools and Software for Graphing
Excel
Excel is a familiar ally for many analysts, and its role in graphing cannot be understated. With its user-friendly interface and powerful capabilities, Excel allows you to create a range of visual representations swiftly. Investors often praise Excel for its capacity to handle large datasets, making it a favorite for constructing time series graphs. Its chart functions are straightforward. You can easily select your dataset and plot it in a visually appealing manner.
One unique feature of Excel is its conditional formatting. This tool can be particularly useful for highlighting significant data trends, such as sudden price spikes or drops. However, it’s worth noting that while Excel is widely accessible, it may struggle with larger datasets as effectively as more robust software options could.
Python Libraries
On the other side of the spectrum, Python libraries like Pandas and Matplotlib present a more advanced option for graphing. Particularly, Pandas offers powerful data manipulation capabilities that set it apart. When combined with Matplotlib, analysts can create highly customized time series graphs that fulfill specific needs.
What stands out about Python libraries is their flexibility. You can automate graphing processes or analyze real-time data trends, which is especially helpful in fast-paced financial environments. However, there might be a learning curve for those not already familiar with programming, which can be a barrier for some users.
Statistical Software
Lastly, specialized statistical software such as R or SPSS comes into play. These programs provide extensive features for data analysis and visualization. They are exceptional in generating time series graphs that are both precise and aesthetically pleasing. A key characteristic of this software is the ability to handle complex models, enabling a deeper dive into financial analytics.
One unique selling point of statistical software is their built-in functions for time series analysis. This allows users to easily apply various models and see resultant graphs in a streamlined fashion. However, the trade-off here can be the cost or the need for extensive training to fully leverage their capabilities.
Graph Design Principles
Once the data is collected and the tools are selected, the next step is adhering to essential graph design principles. A well-constructed time series graph not only represents data but also tells a story. Key considerations include:
- Clarity: Ensure each element in the graph is easily distinguishable. Use contrasting colors appropriately and label axes clearly.
- Scale: It’s vital to select a proper scale for your y-axis, as misleading scales can distort perceptions.
- Legends and Labels: These should be comprehensive but succinct, ensuring that the viewer does not struggle to decode what the graph represents.
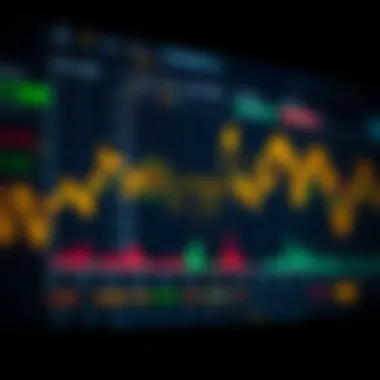
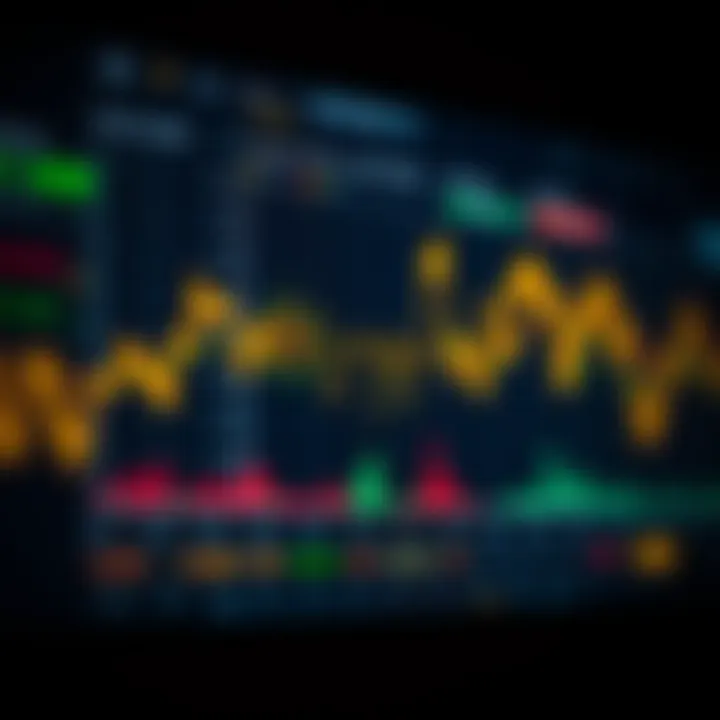
Incorporating these principles while constructing a time series graph can greatly enhance comprehension and engagement from investors to analysts.
Successfully constructing and interpreting time series graphs can empower stakeholders in their financial decision-making processes, ultimately leading to more informed investment strategies.
Analysis and Interpretation of Time Series Graphs
Understanding how to analyze and interpret time series graphs is like holding the compass in a vast ocean of data. It doesn’t just tell you where you've been but also helps you navigate where you could be heading. Each data point plotted over time is not merely a number; it tells a story rich with information about past performances, allowing analysts to spot trends and make informed predictions about the future. In finance, this knowledge proves crucial when developing strategies or assessing risk.
When you grasp the nuances involved in the analysis of time series graphs, it opens a portal to a wealth of insights. For investors and financial advisors, identifying pronounced trends may guide decisions on when to buy or sell. Financial analysts, meanwhile, utilize this analytical lens to draw conclusions, all based on comprehensive data narratives woven into the graphs.
However, the spyglass into this world comes with its set of complexities. Analysts must always consider the context of the data. Variations might arise from seasonal factors, anomalies, or economic conditions. Understanding these elements is just as important as the analysis itself because it ensures that interpretations aren't skewed by misleading outliers or cyclic noise.
Identifying Trends
Trends, in essence, are the long-term movements evident within time series data. To some extent, recognizing these trends can be broken down into three categories: uptrends, downtrends, and sideways trends. Identifying a trend involves examining historical data: do the data points reflect a steady increase, a decrease, or a consistent plateau over time?
Using visual cues from a time series graph, such as line slopes and proximity of points, can reveal much about these trends:
- Uptrends are evident when there's a series of higher highs and higher lows. This signals growing investor confidence.
- Downtrends appear as lower highs and lower lows, a potential warning sign of waning market interest.
- Sideways trends suggest a period of consolidation, which can either precede significant movements or indicate market indecision.
Understanding these trends isn't just about reading graphs; it involves strategic thinking regarding asset allocation and market timing.
Detecting Seasonality
Upon diving deeper into the timeline of financial data, one often stumbles upon seasonal patterns. These are predictable fluctuations that occur at regular intervals, influenced by factors like economic cycles, weather, or consumer habits. For example, retail sales often rise during holiday seasons, while agricultural outputs may sway with seasonal harvests.
Detecting seasonality in time series graphs requires keen observation:
- Look for consistent peaks and troughs at specific intervals.
- Compare the same periods across multiple years to confirm that the patterns aren’t flukes but rather reliable occurrences.
Understanding seasonality aids in projecting future performance since it allows analysts to adjust their strategies to bypass or capitalize on expected fluctuations. By anticipating these cycles, financial professionals can plan better for peak and off-peak periods, optimizing their operations and investments accordingly.
Recognizing Anomalies
Recognizing anomalies—those sudden, unexpected spikes or drops in data—is vital. Financial markets can be a volatile beast, often influenced by news events, economic shifts, or company-specific news. These anomalies can skew perceptions of performance and must be cautiously interpreted.
When interpreting time series graphs, look for:
- Outliers that are significantly different from the trend. These may warrant further investigation to discern whether they’re due to genuine shocks or irrelevant noise.
- Breaks in trends, such as sharp declines or sudden surges, can indicate major transitions likely influenced by economic events or industry developments.
Addressing these anomalies requires a blend of caution and analytical vigor—other relevant indicators, such as trading volume or news reports during these periods, can provide context to help distinguish between fearful reactions and valid shifts in market sentiment.
"In the intricate dance of financial data, understanding where the steps get shaky can preserve your footing on solid ground."
As analysts gain experience in analyzing time series graphs, the ability to detect trends, understand seasonal influences, and recognize anomalies becomes second nature. Ultimately, this analysis fosters a more robust understanding of the financial landscape, empowering investors and analysts alike to make better-informed decisions.
Advanced Techniques in Time Series Analysis
Advanced techniques in time series analysis are crucial because they elevate the analytical process beyond simple observation. These methods bring depth and precision to the understanding of temporal data, which is especially indispensable for investors and financial analysts looking to make educated decisions. Rather than merely recognizing trends or patterns, these techniques enable analysts to enhance prediction accuracy and resilience in rapidly changing financial landscapes.
When dealing with time series data, practitioners often grapple with a multitude of challenges, including noise and irregular fluctuations. Advanced techniques help in dissecting these components and refining data interpretation. This section focuses on two important aspects: smoothing methods and decomposition techniques.
Smoothing Methods
Smoothing methods are widely employed in time series analysis to create a clearer picture of long-term trends by reducing noise inherent in data. This is crucial for making informed decisions, as investors can seize opportunities that might otherwise go unnoticed amid short-term fluctuations.
Moving Averages
Moving averages serve as one of the simplest yet highly effective smoothing techniques. The primary feature of moving averages lies in its capacity to smooth out short-term variations while highlighting longer-term trends. By averaging data points over a specific time period, it becomes easier to discern where the data might be heading instead of being distracted by daily or weekly spikes.
A key benefit of moving averages is their ability to adapt to new data over time. However, these averages aren't without their drawbacks. The lag in response to sudden market changes can lead to missed opportunities. Investors need to be cautious, as relying solely on moving averages may lead to complacency.
"Moving averages are like a lighthouse in a storm; they guide traders but may not capture every ripple of the waves around them."
Exponential Smoothing
Exponential smoothing is another powerful technique that offers a tailored approach to forecasting. In contrast to simple moving averages, exponential smoothing gives greater weight to recent observations, making it highly responsive to changes in trends. This is particularly useful in financial markets where conditions can shift quickly.
The unique selling point of exponential smoothing is its ability to provide faster insights with less lag compared to other smoothing techniques. However, this responsiveness comes with its own set of challenges. For instance, while it helps detect trends faster, it may also amplify short-term fluctuations, leading to potential misinterpretation.
Decomposition of Time Series
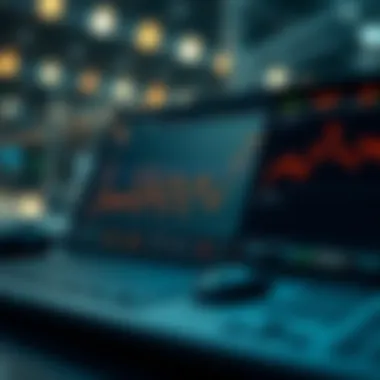
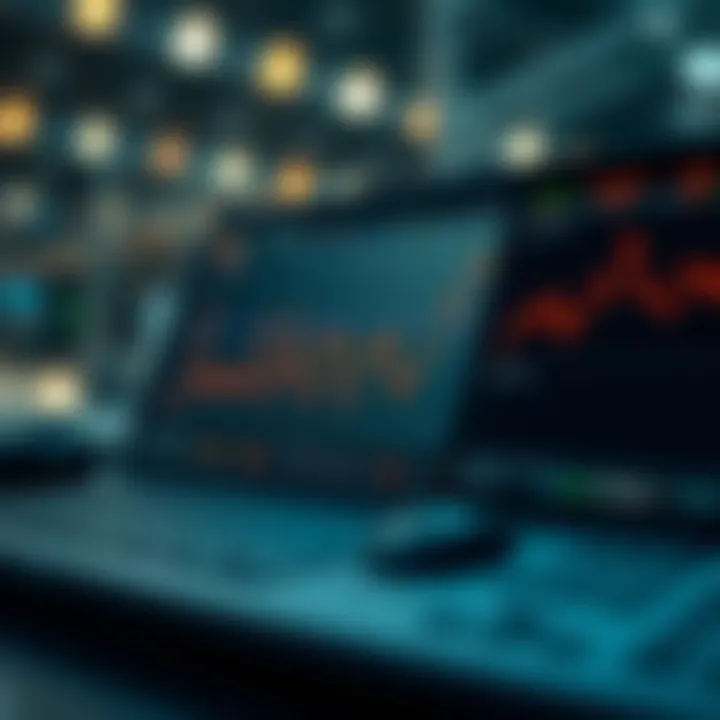
Decomposition is instrumental in breaking down time series data into its fundamental components: trend, seasonality, and residuals. This process enhances understanding by allowing analysts to focus on specific aspects of the data rather than the noise that can cloud interpretation. By understanding these components, investors can refine their strategies and adapt to emerging trends more effectively.
A comprehensive understanding of decomposition facilitates tailored analyses. For example, if a company’s sales data exhibits seasonal spikes during the holiday season, the data can be adjusted, allowing for better predictions and strategic planning throughout the year.
These advanced techniques in time series analysis not only bolster data interpretation but also enhance financial decision-making capabilities for investors. Engaging with these methods is a step toward developing a more nuanced and informed approach to financial analysis.
Applications in Financial Decision Making
In the realm of finance, time series graphs serve as vital tools for decision-makers. Analyzing historical data through these graphs offers crucial insights that can help shape investment strategies and mitigate risks. Understanding how to interpret these visuals can empower investors, financial advisors, and analysts to act based on informed expectations rather than guesswork.
Investment Strategy Development
Investment decisions are often a moving target, influenced by market fluctuations and economic conditions. Time series graphs provide a straightforward way to visualize and analyze these changes. Identifying trends in time series data, such as stock prices or interest rates, can guide investors to make strategic choices that align with anticipated market behavior.
When developing an investment strategy, consider the following key points:
- Trend Analysis: By visualizing historical price movements, investors can identify upward or downward trends. This enables them to time their entry and exit points effectively.
- Pattern Recognition: Identifying recurring patterns in the data, such as seasonal spikes, can help investors anticipate future movements. For instance, retail stocks often rise during holidays.
- Historical Benchmarks: Comparing current performance against historical data allows for performance evaluation, helping investors to gauge whether certain assets are underperforming or outperforming on their historical metrics.
For example, let’s say an analyst is studying the stock price of Company A over several years using a time series graph. Through meticulous examination, they might discover a pattern indicating that the stock price tends to increase every spring. This insight, gleaned from a well-constructed graph, can guide the analyst’s recommendations, steering clients to potentially lucrative investments during that season.
Risk Management Applications
Risk management is another critical area where time series graphs shine. They enable professionals to navigate the choppy waters of financial uncertainty by providing data-backed views of potential risks and rewards.
Some notable aspects include:
- Volatility Analysis: Charts can reveal periods of high volatility or stability. Understanding these can help firms take preventive measures when the market shows signs of volatility.
- Scenario Analysis: By simulating various market conditions and visualizing potential outcomes, firms can better prepare for different risk scenarios, allowing them to formulate contingency plans.
- Decision Making: Well-crafted time series graphs simplify complex data, making it easier for decision-makers to grasp critical information quickly. An efficient presentation can often support urgent calls to action in a crisis.
For instance, consider a hedge fund manager monitoring market volatility through a time series graph. By noticing patterns of spikes in volatility, they can hedge against potential downturns effectively and reallocate resources to minimize exposure.
“Graphs help simplify the complexities of financial data, transforming numbers into narratives that tell us what’s happening on the ground.”
Challenges and Limitations
In the realm of financial analysis, time series graphs are powerful tools for revealing trends, seasonality, and potential anomalies in data. However, like any analytical method, they come with their own set of challenges and limitations that can affect their accuracy and utility. Understanding these issues is vital for investors, financial advisors, and analysts as they navigate the complexities of financial markets. This section aims to shed light on specific challenges, ensuring readers approach their time series analyses with a comprehensive understanding of what may impact their findings.
Data Quality Issues
The integrity of time series analysis hinges upon the quality of the data fed into the system. If the data collected is flawed, whether due to measurement errors, biases in data collection methods, or outdated information, the resulting analysis can be compromised. Financial data is often subject to revisions, particularly when it comes to quarterly earnings or economic indicators. Moreover, data may also be missing or contain outliers that skew results. Inaccuracies might arise not only from the raw data but also from the methods employed to consolidate, clean, and interpret that data.
Here are some common data quality issues:
- Inconsistency: Different sources may report varying figures. For example, two financial news outlets reporting on a stock’s price might display slightly different numbers due to timing variability.
- Completeness: Missing elements in the dataset can introduce noise. Imagine trying to identify trends in consumer spending if certain months of sales data are omitted, leading to skewed patterns in your graph.
- Outliers: These are data points that deviate significantly from the rest of the dataset. They could signal an anomaly or reflect a data entry error, both of which could distort trend analysis.
Investors should always validate the data they use, cross-referencing multiple reliable sources when possible. This step helps minimize the risk of basing critical decisions on unreliable charts.
Model Overfitting
Another significant challenge in time series analysis is the danger of model overfitting. Overfitting occurs when a model captures noise rather than the underlying trend within the data. This issue is particularly prevalent in complex financial datasets, where models can become so tailored to past data that they fail to generalize to new, unseen data. For instance, if a model is trained to recognize subtle fluctuations within a stock’s price but doesn't account for broader market shifts, it may perform exceptionally well on historical data but poorly in future projections.
Key points regarding model overfitting include:
- Complexity vs. Simplicity: A model that is too complex can lead to overfitting, while overly simplistic models may fail to capture essential elements of the data. Striking the right balance is crucial.
- Validation Techniques: Using techniques like cross-validation helps assess the robustness of a model. By dividing a dataset into training and testing sets, analysts can better judge whether a model is able to generalize effectively.
- Regularization Methods: Incorporating regularization can help keep models in check, discouraging them from fitting noise and promoting a more generalizable outcome.
Ultimately, systematically addressing the challenges and limitations of time series analysis is imperative for crafting reliable and informative conclusions. Awareness in these areas enables analysts to enhance their methodological approaches, leading to better-informed investment decisions.
Future Trends in Time Series Analysis
As the realm of financial analysis evolves, the use of time series graphs is increasingly intertwined with innovative technologies. Understanding the future trends in time series analysis is crucial for investors, financial advisors, students, entrepreneurs, and analysts alike. These trends not only enhance the capability to analyze historical data but also optimize how insights are generated for decision-making. The speed of change in data technology means that trends must be closely monitored to maintain a competitive edge.
Integration with Machine Learning
The integration of machine learning into time series analysis is a game changer. Traditional methods often rely on statistical formulas that can be limiting when faced with vast datasets. Machine learning algorithms, however, can adapt and improve over time, learning from new data without explicit reprogramming. Here are some key points to consider:
- Flexibility: Machine learning models can adjust to changes in data patterns quickly, making them crucial for financial markets where conditions can shift in a blink.
- Predictive Power: Algorithms like recurrent neural networks (RNNs) or long short-term memory (LSTM) networks can effectively capture temporal dependencies, offering enhanced predictive capabilities than standard methods.
- Automation: Automating the detection of trends or anomalies within time series can save countless hours of manual analysis, allowing professionals to focus on interpreting results instead of data wrangling.
"Machine learning transforms time series analysis from a static approach to a dynamic, continuously evolving process."
Enhanced Predictive Capabilities
The future of financial analysis hinges on the predictive capabilities of time series data. As technology progresses, tools that employ advanced machine learning techniques are set to become more sophisticated. These improvements can significantly influence financial decision-making, as deeper insights can be gleaned from both historical data and real-time inputs. Consider the following benefits:
- Increased Accuracy: More accurate predictions mean that investors can make more informed choices, reducing the likelihood of costly errors.
- Scenario Analysis: Enhanced modeling allows for better testing of various financial scenarios, leading to robust risk management strategies.
- Real-time Insights: With enhanced processes, analysts can obtain instantaneous insights, a necessity in today's fast-paced financial environment.
Efforts to refine the algorithms and models will continue, ultimately leading to practices that blend human oversight with automated insights. It's a promising frontier that deserves attention—the ability to perceive patterns and forecast financial movements will redefine investment strategies.
By staying abreast of these emerging trends, professionals in finance can leverage time series analysis to navigate the complexities of the market and make decisions that stand the test of time.