Understanding Z Scores and T Scores in Statistics
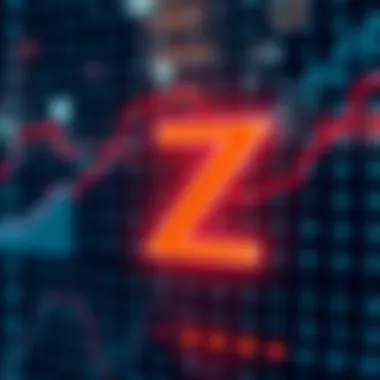
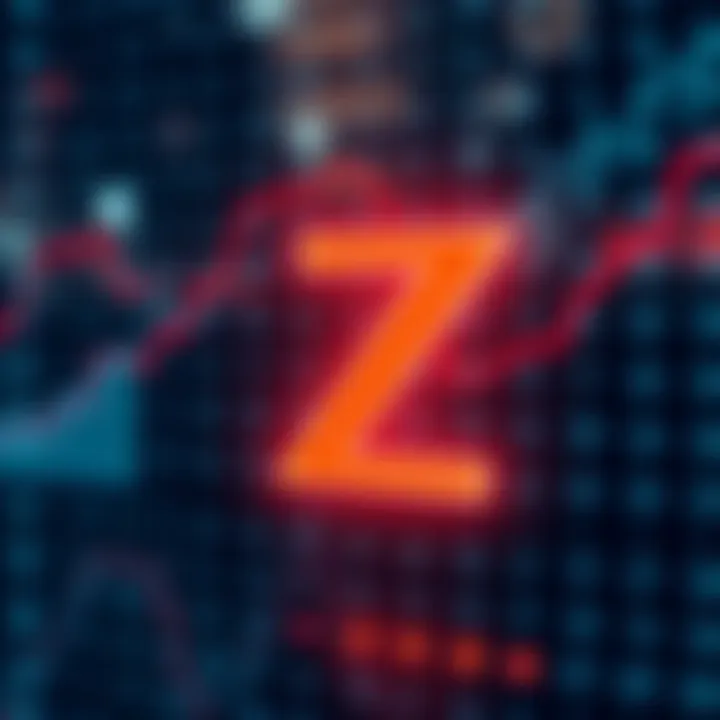
Intro
In the realm of statistics, numbers tell stories. When you peel back the layers of data, Z scores and T scores pull out crucial insights from the noise. Understanding these two statistical measures is not just for the math enthusiasts; itβs a skill that can empower investors, financial advisors, and analysts alike. This article aims to shed light on these concepts, distinguishing their definitions, applications, and contexts where they shine.
Z scores and T scores are essential when analyzing data sets, especially when assessing how far an individual data point lies from the mean. While both are used for standardizing scores to interpret relative positioning, they serve distinct purposes depending on the sample size and the normality of the data. A clearer grasp of these measures can enhance your statistical decision-making abilities and foster deeper analysis in both academic research and real-world scenarios.
Preamble to Statistical Measures
Statistical measures are crucial tools that provide insight into data sets, aiding in the interpretation and application of data across various fields. By understanding these measures, analysts can make informed decisions based on real evidence rather than mere assumption. When one gets down to it, every number tells a story, and statistical scores help unveil the narrative hidden within the data.
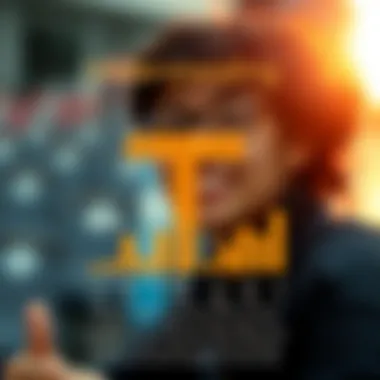
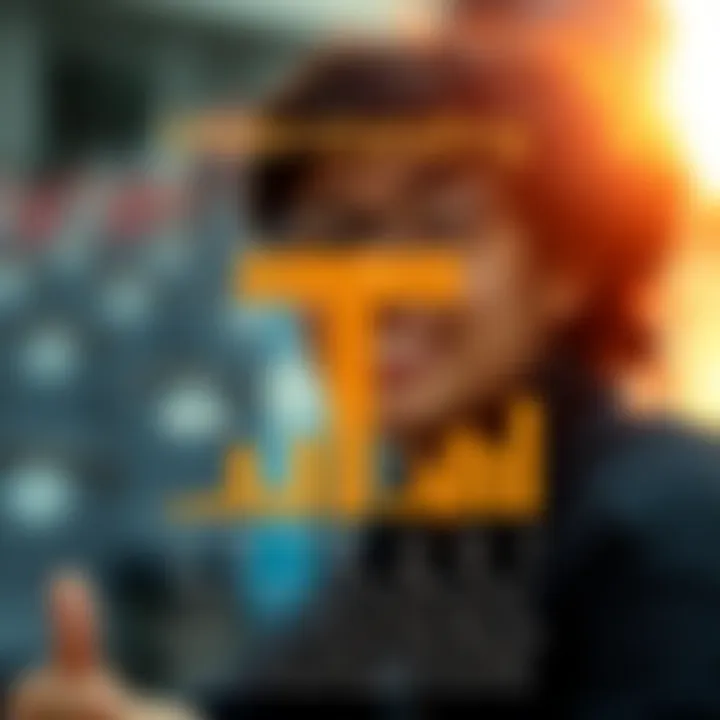
The Purpose of Statistical Scores
Statistical scores, such as Z scores and T scores, serve several essential purposes in data analysis. First and foremost, they help standardize data points, making it easier to compare values from different datasets. For example, if you're looking at scores from two different classes on a standardized test, simply knowing the raw scores may not tell the full story. A Z score tells you how far a particular score is from the mean, expressed in standard deviations. This quantification allows for apples-to-apples comparisons, even when the average scores differ widely between groups.
Beyond normalization, these scores enable hypothesis testing. In practical terms, they assist researchers in determining whether certain outcomes are statistically significant. By analyzing Z and T scores, analysts can ascertain whether the results of an experiment or study are due to chance or are genuinely reflective of underlying trends.
Importance in Data Analysis
In the realm of data analysis, the significance of understanding Z scores and T scores cannot be understated. They are not just academic concepts; they have real-world applicability. Investors glance at scores to analyze market behaviors. Students use these scores to gauge their academic performance, while researchers lean on them to validate their findings.
Moreover, statistical scores aid in identifying outliers. By leveraging Z scores, one can quickly pinpoint data points that are, for example, three standard deviations away from the mean. This ability to detect anomalies is invaluable in finance, where spotting fraudulent transactions early can save organizations from catastrophic losses.
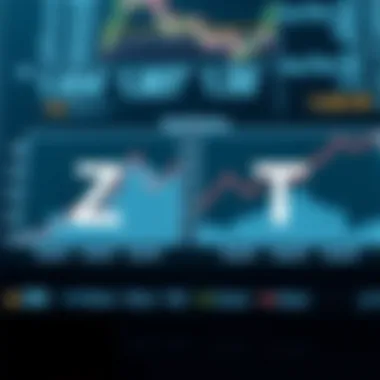
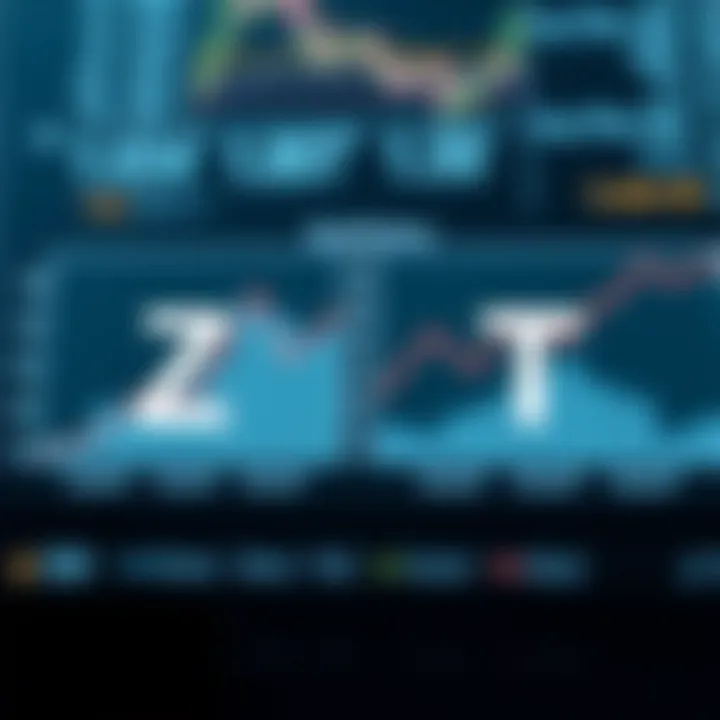
- Precision in Analysis: Enhanced accuracy leads to better decision-making.
- Accessibility of Data: Standardized scores provide a common language for comparison.
- Efficient Communication: They help convey complex information in a digestible form for stakeholders.
Z Scores Explained
Understanding Z scores is crucial for anyone diving into data analysis or exploring the world of statistical inference. These scores provide a way to express how far away a data point is from the mean, measured in terms of standard deviations. By applying this concept, analysts can identify how typical or atypical a data point is within a given dataset. Z scores are particularly useful in contexts involving normal distributions, where understanding relative positioning becomes key.
Definition of Z Scores
A Z score, also known as a standard score, quantifies the distance of a data point from the mean of the dataset, expressed in terms of standard deviations. The formula for calculating a Z score is:
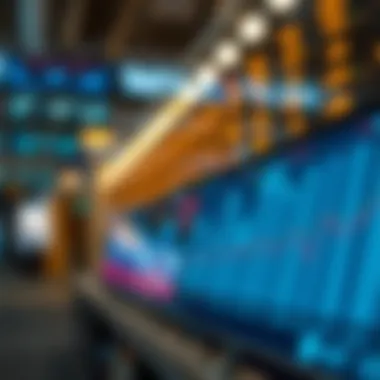
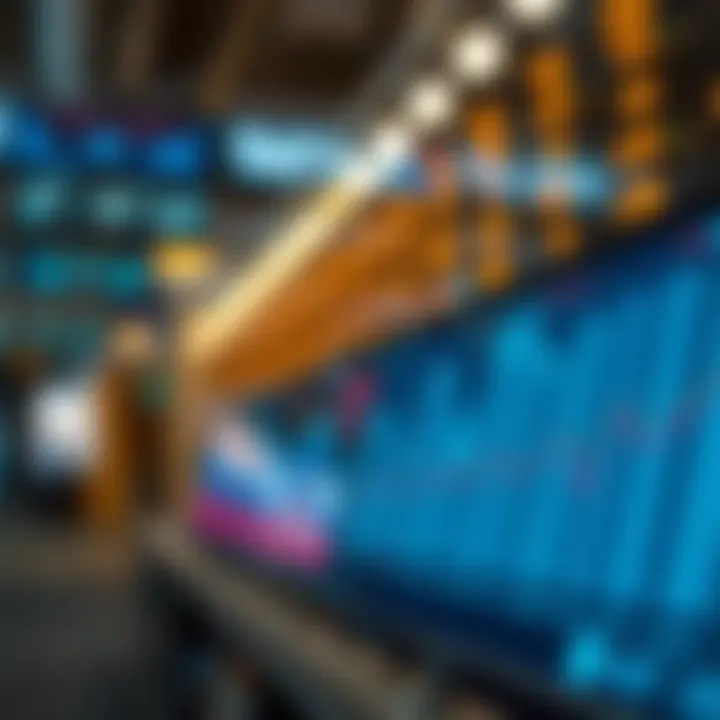
where
- (X) is the value of the data point,
- (\mu) represents the mean of the dataset,
- (\sigma) is the standard deviation.
For instance, if a student's test score is 85, the class mean was 75, and the standard deviation is 10, the Z score would be 1. This means the student's score is one standard deviation above the mean, illustrating their performance relative to their peers.
Formula for Calculation
To calculate a Z score accurately, follow these steps:
- Determine the Mean ((\mu)) - Add up all the values in your dataset and divide by the number of values.
- Calculate the Standard Deviation ((\sigma)) - Measure the variability of the dataset.
- Subtract the Mean from Your Data Point ((X - \mu)) - This gives you the deviation of the data point from the mean.
- Divide by the Standard Deviation - This gives you the Z score, indicating how many standard deviations away the point is from the average.
plaintext Z Score Calculation Example: Data Point (X) = 80 Mean (Β΅) = 70 Standard Deviation (Ο) = 5
Z = (80 - 70) / 5 = 2